article
The Power of Partnership: Accelerating Healthcare's AI Revolution Through Data Quality Improvement & Integration
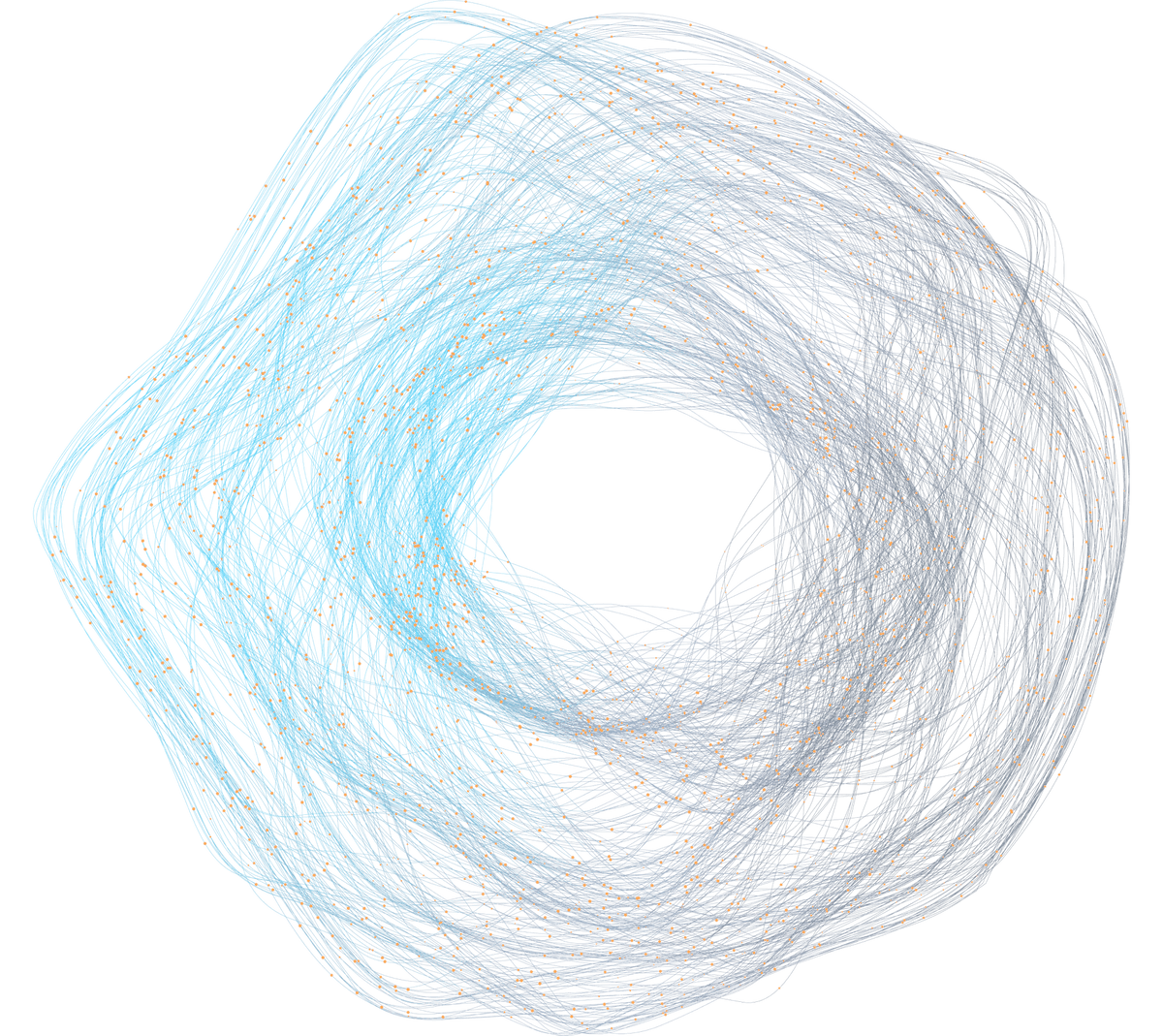
SECTIONS
The Critical Foundation: Data Quality in Healthcare AI
The healthcare industry stands at the cusp of a transformative era, driven by the immense potential of artificial intelligence (AI). From enhancing diagnostic accuracy and personalizing treatment plans to streamlining operational efficiencies, AI promises to revolutionize patient care and healthcare delivery. However, the realization of this promise hinges critically on the quality of the data that fuels these intelligent systems. The healthcare sector grapples with unique challenges in achieving and maintaining the high standards of data quality necessary for effective AI implementation.
The initial excitement surrounding Al in healthcare must be grounded in a clear understanding of the fundamental role data quality plays. Al algorithms are essentially pattern recognition engines. If the data they are trained on contains errors, inconsistencies, or is incomplete, the resulting Al outputs will inevitably be flawed, potentially leading to detrimental consequences in clinical settings. Therefore, a strong emphasis on data quality is not merely a preliminary step but an ongoing necessity for the responsible and impactful deployment of AI in healthcare.
Navigating Healthcare's Complex Data Landscape
Furthermore, the sheer scale and diversity of healthcare data, while presenting significant opportunities for AI-driven insights, simultaneously amplify the complexities associated with ensuring data quality. Healthcare data originates from a multitude of sources, including electronic health records (EHRs), medical imaging systems, wearable devices, and administrative platforms. This data comes in various formats, both structured and unstructured, making it a significant undertaking to aggregate, cleanse, and standardize it to a level suitable for reliable AI applications.
Breaking Down Data Silos: A Healthcare Imperative
One of the primary obstacles to achieving the necessary data quality in healthcare is the pervasive issue of data silos. These isolated systems, which store data independently, hinder a comprehensive understanding of the patient or member and impede the seamless flow of information across the healthcare ecosystem. The ramifications of these silos are far-reaching, leading to incomplete patient histories, delays in diagnosis and treatment, fragmented care coordination among providers, increased healthcare costs due to redundant procedures, and a limited ability to identify critical patterns in patient populations. Studies indicate that a significant majority of healthcare executives recognize data silos as a major barrier to effective analytics. It also impacts the bottom line when errors in data prevent the clean processing of claims.
Beyond the fragmentation of patient records, data silos also contribute to inconsistencies in data formats and coding standards across different systems. This lack of uniformity further degrades the overall quality of data, making it challenging to integrate and analyze information accurately. For instance, the same clinical measurement might be recorded using different units or terminologies in disparate systems.
The Promise and Challenge of Healthcare Interoperability
To overcome these challenges, healthcare organizations must prioritize interoperability, defined as the ability of different information systems, devices, and applications to access, exchange, integrate, and cooperatively use data in a coordinated manner. Achieving seamless interoperability offers numerous benefits, including enhanced coordination of patient care across different providers and settings, improved patient safety through timely access to complete medical histories, increased operational efficiency by streamlining administrative tasks and reducing redundancies, and empowering patients or members with access to their own health information.
However, realizing true interoperability is not without its hurdles. These include the lack of universal data standards across different EHR systems, the persistence of data silos and limited communication between systems, concerns surrounding patient privacy and security in data sharing, the often-substantial costs associated with system integration, and resistance to change among healthcare professionals who may face workflow disruptions.
While standards like HL7 FHIR are crucial for facilitating data exchange by establishing standard protocols, achieving widespread interoperability necessitates a concerted effort that extends beyond technology implementation to encompass organizational culture and commitment.
Introducing Coperor: A Unified Approach to Healthcare Data Management
This is where Gaine emerges as a strategic partner, offering Coperor™ Health Data Management Platform (HDMP), meticulously designed to unify and control the complex landscape of healthcare data. At its core, Coperor provides robust Master Data Management (MDM) capabilities, coupled with strong data governance frameworks and seamless interoperability solutions. By effectively breaking down data silos, Coperor creates a single, longitudinal view of critical healthcare information, including patient, provider, claims, and clinical data. The platform not only supports traditional interoperability standards like HL7 and FHIR but also extends them with a sophisticated health data model, ensuring both efficient data exchange and the preservation of semantic context for advanced analytics.
Coperor's unified approach distinguishes it by offering a centralized solution to the multifaceted challenges of data quality and interoperability in healthcare, moving beyond siloed, point-based solutions. Furthermore, its emphasis on maintaining the semantic meaning of data ensures that AI algorithms can accurately interpret and leverage the information, leading to more reliable and actionable insights.
Enhancing Data Accuracy: The Foundation of Effective AI
Partnering with Gaine empowers healthcare organizations to significantly enhance their data accuracy, a cornerstone of effective AI. Coperor's MDM capabilities, which include advanced matching, merging, and data cleansing functionalities, work to eliminate errors and inconsistencies across disparate data sources. Features such as cell-level survivorship and the automation of complex business rules further ensure the ongoing integrity of the data. Given the profound impact of inaccurate data on patient safety and the reliability of clinical decision-making, Coperor's ability to provide a single, trusted source of truth for healthcare data is invaluable. This minimizes the risk of Al algorithms being trained or operating on conflicting or erroneous information, thereby substantially improving their predictive accuracy and overall effectiveness.
Achieving Comprehensive Data Completeness
Ensuring data completeness is another critical area where Coperor provides significant value. The platform's ability to integrate diverse data sources enables a comprehensive and holistic view of patient or member information. Access to complete patient data, including medical history, diagnoses, treatments, and other relevant factors, is paramount for accurate diagnoses and the development of truly personalized treatment plans. By consolidating data from various systems, Coperor ensures that AI algorithms have access to the full spectrum of a patient's health journey, leading to more informed and impactful AI applications.
Standardizing Data for Enterprise-Wide Consistency
Maintaining data consistency across the healthcare enterprise is facilitated by Coperor's robust data governance policies and standards. The platform's reference data management capabilities play a crucial role in standardizing medical terminologies and coding systems, addressing the significant challenges posed by inconsistent data formats and terminologies. By ensuring uniformity in data representation, Coperor enables AI algorithms to process and analyze information in a standardized manner, significantly reducing the potential for errors and enhancing the reliability of AI-driven insights.
Delivering Real-Time Data for Timely Healthcare Insights
Furthermore, Coperor's architecture is designed for real-time data delivery across hybrid and multi-cloud environments. The timeliness of data is crucial for many critical healthcare applications, such as the early detection of diseases, real-time monitoring of patient conditions, and proactive intervention strategies. Coperor's ability to provide near real-time access to data ensures that Al algorithms can leverage the most current information available, leading to more timely and relevant insights that support clinical decision-making and improve overall operational efficiency.
Simplifying AI Development and Deployment
By providing a clean, consistent, and comprehensive data foundation, Coperor significantly simplifies the development and deployment of AI models in healthcare. This unified platform abstracts away the complexities typically associated with data integration and quality management, allowing healthcare organizations to focus their valuable resources on the creation and implementation of innovative AI applications tailored to their specific needs.
Powering Diverse AI Use Cases in Healthcare
Coperor's robust data management capabilities provide the essential fuel for a diverse range of Al use cases in healthcare, including enabling personalized treatment plans based on individual patient data, facilitating predictive analytics for disease risk and patient outcomes, streamlining efficient administrative tasks such as claims processing and appointment scheduling, and more.
Accelerating Time to Value with Healthcare-Specific Solutions
Moreover, Coperor's healthcare data model and embedded business rules significantly accelerate the deployment of data solutions and AI initiatives. Our agile approach allows organizations to realize business value incrementally, without being overwhelmed by the complexities of documenting an entire enterprise data landscape upfront.
By offering a data platform specifically designed for the healthcare industry, Coperor dramatically reduces the time and effort traditionally required to prepare data for AI, enabling organizations to achieve the transformative benefits of AI faster and more efficiently.
Partner with Gaine to Transform Healthcare Data
The convergence of Al's potential in healthcare and the critical need for high-quality data presents a unique opportunity for organizations ready to innovate. Gaine stands as the ideal partner to navigate this landscape, offering a proven platform to address the intricate challenges of data quality and interoperability. By partnering with Gaine, healthcare organizations can unlock the full power of their data, accelerate their AI initiatives, and ultimately deliver superior patient or member care.
We invite you to connect with us for a meeting to explore your specific needs and discover how Coperor can empower your organization to achieve its data quality and Al aspirations. Let's transform healthcare together, one data point at a time.
Please reach out to scott.yungling@gaine.com to share your thoughts.